Potential for high autonomy and increased efficiency
- Automation
- Intralogistics
- Robotics
- 11.2.2025
- Reading Time: {{readingTime}} min
- Share Article
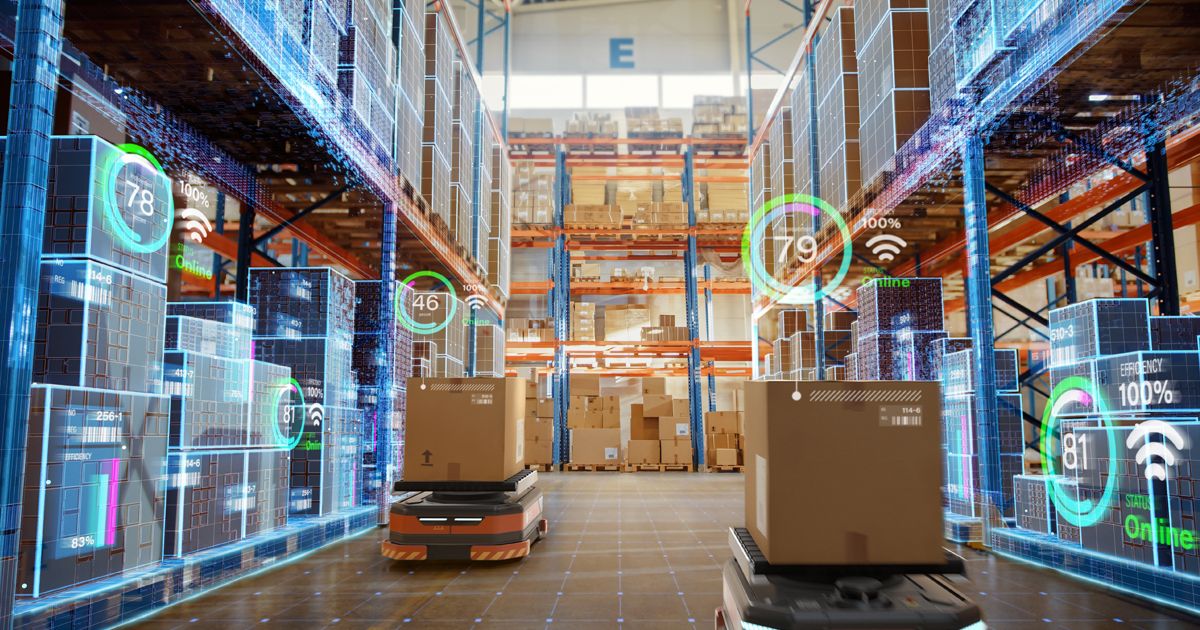
Contents
In the logistics industry in particular, highly autonomous mobile robots offer enormous opportunities to make processes more efficient and optimize them in the long term. According to the World Robotics 2024 Service Robots Report, logistics is currently the largest application area for mobile robots, and this market is growing rapidly. However, despite this potential, many robotic solutions are still in their early stages, especially in terms of autonomy.
Top 5 areas of application for mobile robots
The current situation: transportation and simple tasks
Today, mobile robots in logistics primarily perform transportation tasks between fixed points, with their activities often limited to simple tasks such as handing over parcels or moving materials. These robots often work on predefined, fixed routes and do not require complex decision-making or adaptation to their environment. Typically, these systems are equipped with basic control technology, such as sensors for lane guidance and bumpers, that enable them to follow simple routines and to stop safely when they come into contact with an obstacle, without causing any damage.
Examples of such solutions are Automated Guided Vehicles (AGVs), which are used in large warehouses or fixed production lines. These systems use magnetic tapes or QR codes as landmarks on the floor for orientation and perform tasks such as transporting goods from one point to another. However, such systems are not very flexible because they can only function in clearly defined and controlled environments, such as production lines or automated warehouses – in other words, they only work effectively where processes have little variability.
According to the World Robotics 2024 Service Robots Report, most mobile robots currently used in logistics perform repetitive tasks and navigate either via physical markings or virtual routes along predefined processes. Although their potential is high, their autonomy remains limited. However, modern developments in this area are increasingly relying on advanced sensor technologies such as 3D LiDAR (Light Detection and Ranging) or cameras that enable robots to follow a defined route without the need for structural measures. At the same time, these technologies allow the detection and avoidance of obstacles, which is why such systems are often referred to as autonomous mobile robots (AMR).
Highly autonomous mobile robots are the next big step for intralogistics.
The potential for high autonomy: robots with advanced capabilities
In contrast to today's mostly simple transport robots, highly autonomous mobile robots offer far more potential for the logistics industry. Such systems are not only able to scan their environment, but also to make independent decisions to perform complex tasks. In addition to the task of transport, they can, for example, sort products during the journey, label them or prepare them for subsequent process steps. For example, a robot could sort goods by region of destination and place them in the appropriate packaging during transport.
However, for these more advanced applications, mobile robots need advanced navigation systems and artificial intelligence (AI) that enable them to understand and react to dynamic environments.
AI in logistics: the current situation
The use of artificial intelligence in the logistics industry has increased significantly in recent years. While in 2019, just under 15 percent of German logistics companies were working with AI, three years later, 22 percent of German companies were already using artificial intelligence – from demand forecasting and sales planning to transport optimization. This illustrates how dynamically AI technologies are establishing themselves in the logistics industry. [Source: www.transportlogistic.en/industry-insights ]
Technological key components for high autonomy:
SLAM (Simultaneous Localization and Mapping): While SLAM technology does enable simultaneous localization and mapping, in intralogistics robots require a precise position in a known environment as well as efficient navigation. Therefore, mapping is usually done separately and then made available to all similar robots in the network. This optimizes route planning and ensures reliable navigation for the entire fleet.
Deep learning: Advanced AI models enhanced by deep learning allow mobile robots to learn and optimize complex tasks without having to be programmed from scratch for each new situation. This is particularly important when it comes to adapting to new logistics tasks.
An example of deep learning in this context would be “visual recognition and object recognition”: robots require advanced camera systems that are complemented by object recognition algorithms. These technologies enable robots to identify, classify and interact with objects in their environment. AI-based models such as deep convolutional neural networks (DCNNs) have proven particularly effective at accurately recognizing objects to optimize navigation.
With such functionalities, transport routes and times could be used more efficiently, which could lead to significant cost and time savings.
For example, a large logistics company was able to achieve a 225% improvement in picking efficiency by using autonomous mobile robots (see case study in German).
To further increase the efficiency of these technologies, they should be easily integrated into the respective development environment, work together efficiently and remain maintainable.
Andreas Reingruber, Section Manager Intralogistics at KEBA, says: “Highly autonomous mobile robots are the next big step for intralogistics. Their ability not only to navigate but also to make complex decisions in real time will significantly increase efficiency and flexibility throughout the entire supply chain. Innovative, modular platforms enable these technologies to be seamlessly integrated into existing systems.”
Technologies from other industries as a source of inspiration
While the logistics industry is still in its early days when it comes to highly autonomous robots, other sectors are already well advanced and can serve as a model:
Agriculture: agricultural robots perform highly precise tasks such as weeding or harvesting, guided by multispectral cameras and AI-powered image processing technologies. These technologies enable robots to distinguish between different plant species and respond to specific crop needs.
Airports: self-driving vehicles and robots that transport baggage are already operating with extremely high autonomy. These robots use a combination of LiDAR, cameras and AI-driven algorithms to navigate the complex airport environment, analyze passenger flows and avoid obstacles.
Gastronomy: In the gastronomy, robots are used that not only perform simple tasks such as delivering orders, but are also able to recognize the meals ordered and deliver them autonomously. These robots use object recognition and localization technologies to interact with the environment. In Japan, serving robots in restaurants are by no means uncommon. (See the following video )
Object recognition as a key factor for logistics robots
Deep learning algorithms such as YOLO (You Only Look Once) or Faster R-CNN offer a fast and precise way to recognize objects in real time, which is crucial for logistics robots. For example, they can detect the position of pallets, monitor inventory, or even perform quality assurance processes in real time.
In practice, a logistics robot could use YOLOv5 – a further development of the YOLO algorithm for effective object recognition in real time – to recognize objects on a conveyor belt, sort them and automatically forward them to the correct location. This reduces the need for manual intervention and increases efficiency.
Optimization through custom datasets: To further improve the accuracy of object detection, logistics companies should train AI models on their own datasets. These datasets, which are specifically tailored to the environments in warehouses and logistics centers, enable higher precision and customization to the specific needs of the company.
Our white paper, “Training an Object Detection AI Model on a Custom Dataset” (to the download), explains how to develop such models to meet industry needs.
CONCLUSION: The road to highly autonomous logistics
Mobile robotics in logistics is at the beginning of a transformation. To realize the full potential of this technology, companies must not only invest in more powerful hardware, but also implement advanced AI technologies such as SLAM and deep learning for object recognition. These technologies enable robots to perform complex tasks, increasing efficiency across the entire supply chain. Industries such as agriculture, airport operations and hospitality are already showing us how these technologies work in practice – but the importance of AI is also rapidly increasing in the logistics industry.
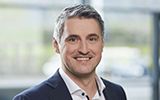